Listen now
The skyrocketing popularity of ChatGPT and other text-to-image generators have shed light on the power of generative AI models. Beyond the several different applications of Generative AI, generative techniques, like GANs and diffusion models, are used to create synthetic data and solve real pain points for enterprises.
Gartner states that by 2024, 60% of data used for the development of AI and analytics projects will be artificially generated, and that, by 2030, it will completely overshadow real data in AI models.
AI requires a massive amount of data to work well and, although organizations generate a tremendous amount of data every day, a large portion of it cannot be used due to regulations or low quality (i.e., data is incomplete, inaccurate, or inconsistent). Hence, synthetic data allows developers to train and test models with a scale, speed, and level of control that is impossible with data collected from the real world, and often for a fraction of the cost.
In this blog, we’ll discuss the practical applications of synthetic data for the enterprise and give our perspective on the market opportunity.
Examples of synthetic data in use today
Synthetic data is, by definition, artificial data generated by Generative AI, rules, statistical models, simulations, random generation, or other techniques to re-create or augment an original dataset. There are numerous applications of synthetic data, so we’ll quickly discuss the need and real-life examples of the three key ones.
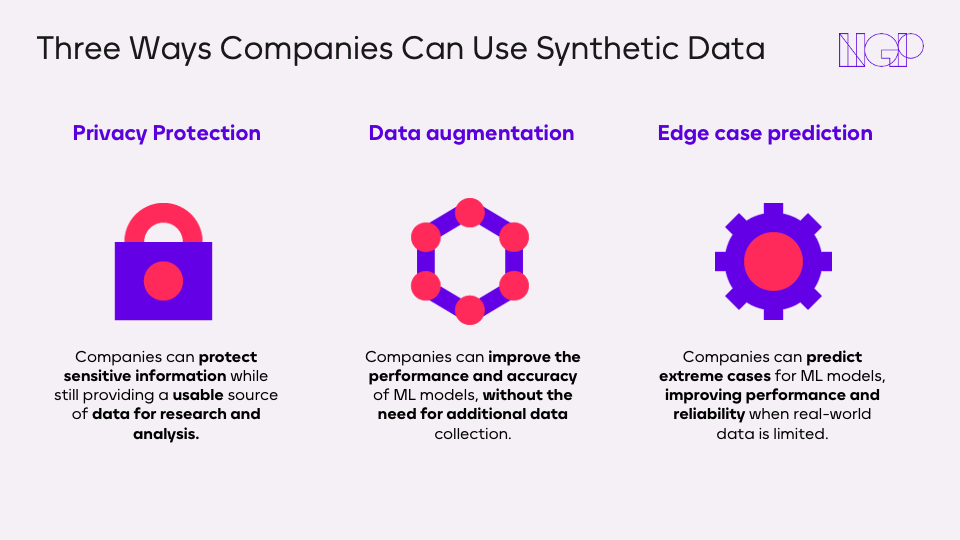
1. Privacy protection: a large bottleneck that organizations face today is the inability to work with their customer or transactional data because it has personally identifiable information (PII) that is heavily protected by regulations. With synthetic data, developers can re-create a fully anonymized and privacy-preserving dataset based on the original data with all statistic characteristics maintained.
As a practical example, Vienna-based Mostly AI recently shared a partnership with Telefonica to synthesize millions of CRM records and unlock 80-85% of customer data in a 100% GDPR-compliant manner. This is huge because companies can now have a better understanding of customer behavior, run predictive analyses, or even monetize this data. This is especially relevant for industries that are strongly regulated such as Financial Services, Insurance, Research & Healthcare, Telecommunications, etc.
2. Data augmentation: as mentioned earlier, there’s not enough good data available, and/or it can be too costly and time-consuming to acquire it. Synthetic data provides a much cheaper and faster alternative to expand the training data set and can also increase the accuracy of AI, such as computer vision and NPL models.
Training robots to make sense of thousands of objects in a dynamic environment can be a painful and long process. Being able to train algorithms based on photo-realistic simulations of objects in different colors, textures, or types without having to collect vast quantities of real-world data can save significant costs. Tel Aviv-based Datagen is a synthetic data company that lets the user fully configure the dataset based on parameters such as identity & demographics, environment & lighting, camera setup, etc.
3. Prediction of edge cases: Synthetic data companies help produce samples of unlikely situations so that ML models learn to recognize them. This is particularly useful in cases where real-world data is scarce or difficult to obtain, such as in the development of autonomous vehicles or medical diagnostic systems. An example is the California-based Parallel Domain platform which is developing thousands of different scenarios to scale perception models and prepare them for the unpredictability of the real world.
Exploring the synthetic data market opportunity
The synthetic data market is growing at a fast pace with several startups offering synthetic data generation tools, platforms, and services. We've seen a variety of business models, and because we believe that synthetic data will become commoditized over time, consumer value will likely come from models that go beyond data generation. We’ve gathered below a non-exhaustive list of relevant startups and open source projects in the space, split by their focus on structured and unstructured data.
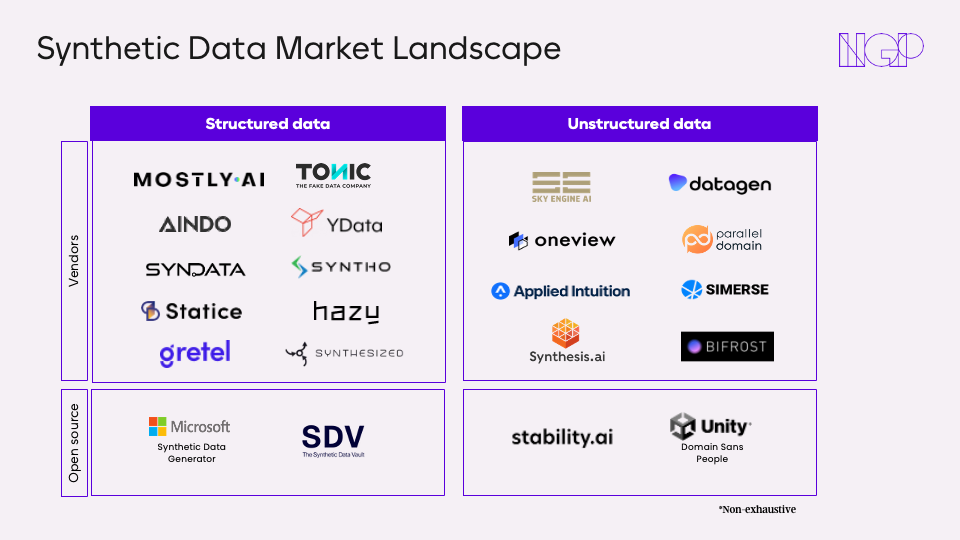
For startups focused on structured data (text, tabular, etc), we see most differentiation coming from an end-to-end approach. Meaning, platforms that i.e. allow customers to perform data transformation, check the quality of the input data, generate their own synthetic datasets, train models, and ultimately be able to integrate with other platforms in the enterprise’s data stack. For example, Seattle-based Ydata is building a data-centric development platform that helps data scientists from data quality visualization and improvement to faster deployment of AI on a single platform. There are many models that could succeed, but overall startups that rely on 3rd party integrations need to guarantee that the software seamlessly integrates with other products while providing a great customer experience.
When it comes to unstructured data (video, image, etc), our view is that the competitive advantage derives from abstracting complexity from the synthetic data generation process. It could be, for instance, by allowing any data scientist or developer to build 3D models and simulations without having a 3D design background. Siemens’ in-house Synth AI software is using a similar approach as they help manufacturers automate machine vision training without the need for experts, by simply uploading CAD data that is then transformed into randomized synthetic images and used to train ML models automatically. Another example is London-based Sky Engine, which is developing a proprietary physics-based rendering engine that can be controlled using a python API and promises to deliver superior image and video quality. In our opinion, this can significantly decrease costs and time to value.
Synthetic data will alter the way AI is developed
Synthetic data is not a new idea but is currently gaining significant traction in practical applications. Obtaining the appropriate data is crucial for AI development, but it can be difficult, costly, and time-consuming. Synthetic data is on the verge of fundamentally altering the way AI is developed, and this shift is expected to have a significant economic impact by driving innovation and unlocking new opportunities across industries.
At NGP, we’re excited about what it’s to come in the rapidly evolving AI space and very much welcome challenging views and comments.
If you have any questions or feedback related to this post, please reach out to fernanda@ngpcap.com.